TMUDCC: Taipei Medical University Dengue Case Corpus
Motivation
Globalization and environmental changes have increased the emergence or re-emergence of infectious diseases worldwide. Regional-wide infectious disease surveillance system collaboration is critical but difficult to achieve due to the different transparency levels of health information sharing systems among countries. ProMED-mail, the most comprehensive expert-curated platform which provides rich outbreak information among humans, animals, and plants from different countries. However, the unstructured text content of the reports makes it difficult to analyze for further applications. In addition, the outbreak of dengue fever in Southeast Asia is a critical issue in infectious diseases worldwide. Therefore, we have come up with an idea to develop an automatic summary of the alerting articles from ProMED-mail, the huge textual data source. In this research, we proposed a text summarization method that uses natural language processing technology to automatically extract important sentences from alert articles in ProMED emails to generate summaries. Through our method, we are able to quickly capture crucial information and make the decision for epidemic surveillance.
Methodology
In our experiments, the performance evaluation metrics included precision, recall, and F1-score. And we used macro-average to compute the average performance.
We proposed a linguistic pattern-infused BiLSTM with attention mechanism neural network for dengue case information extraction. That is, to extract sentences which convey dengue case information from alerting articles from ProMED-mail. We treat dengue case information extraction as a binary classification problem, the goal of this task is to decide whether a certain sentence s expresses dengue case information or not. Our framework consists of four main procedures: text preprocessing, dual-channel BiLSTM, linguistic pattern infusion, and dengue case information classifier.
Finally, the proposed deep neural network-based method can integrate linguistic patterns and latent syntactic features to identify important sentences. The results demonstrate that the proposed method successfully exploits the syntactic, semantic, and context information relevant to epidemiological information on dengue. Consequently, our method outperforms many well-known information extraction methods, as well as achieves 83.6% satisfactory ratings for the summarization of dengue alert articles.
Taipei Medical University Dengue Case Corpus (TMUDCC)
We first extracted Southeast Asia dengue fever alerting articles. Then, we requested three experts to annotate the dataset. All the background of the three annotators is both medical and English professional. The Cohen’s Kappa statistic of the labeling process was 0.895 (Figure 1). This statistic indicates that the inter-observer agreement of our annotated data corpus is reliable. It is worth noting that approximately 80.0% of the crucial sentences are composed of multiple clauses which derive complex syntactic structure.
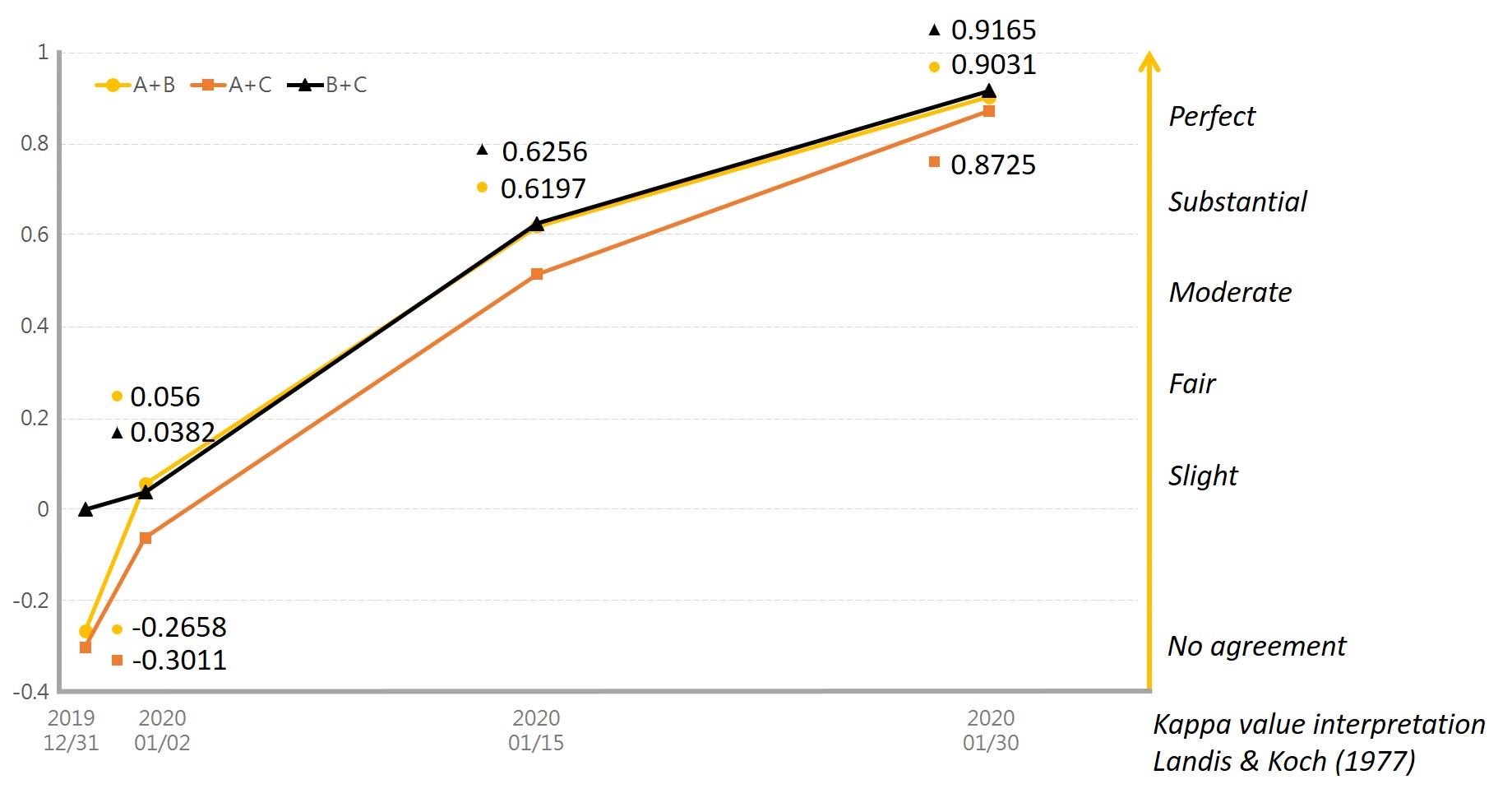
As dengue case information can be narrated in a sequence of clauses, we hereby consider two types of sentences: single-clausal sentences (SC) and multi-clausal sentences (MC). It is worth noting that approximately 80.0% of the key sentences are composed of multiple clauses with a complex syntactic structure (Table 1). Meanwhile, 34.6% of the sentences contain digits which do not convey case information.
Table 1. The statistics of data corpus.
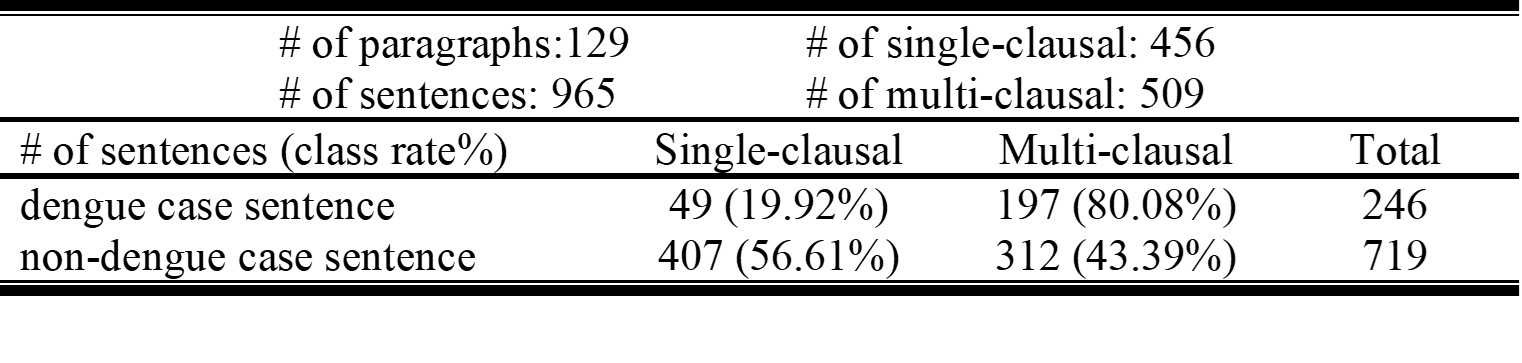